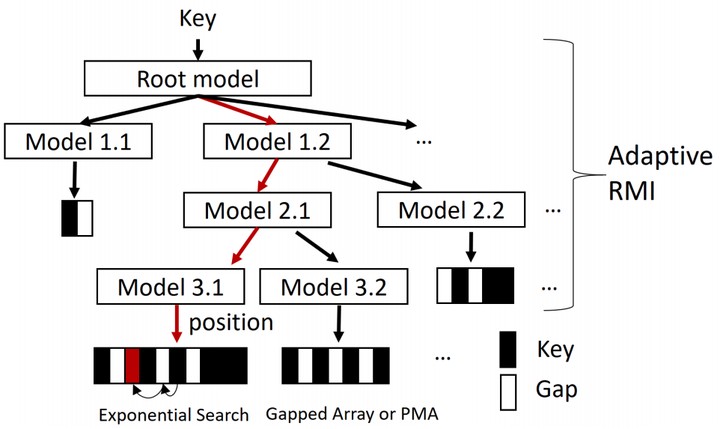
Introduction
ALEX is a new class of learned indexes which addresses issues that arise when implementing dynamic, updatable learned indexes. Compared to the learned index from Kraska et al., ALEX has up to 3000× lower space requirements, but has up to 2.7× higher search performance on static workloads. Compared to a B+Tree, ALEX achieves up to 3.5× and 3.3× higher performance on static and some dynamic workloads, respectively, with up to 5 orders of magnitude smaller index size. Our detailed experiments show that ALEX presents a key step towards making learned indexes practical for a broader class of database workloads with dynamic updates.
Highlight
Publications: SIGMOD 2020 (research)
Collaborators:
MIT: Jialin Ding, Tim Kraska
Microsoft Research: Umar Farooq Minhas, David Lomet, Jae Young Do, Yinan Li, Chi Wang, Badrish Chandramouli, Johannes Gehrke, Donald Kossmann
ETH: Hantian Zhang